Thyroid Disease Risk Assessment Dataset
Public Health & Epidemiology
Related Searches
Trusted By
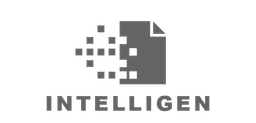
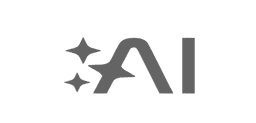
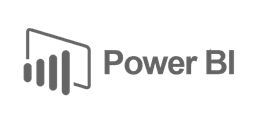
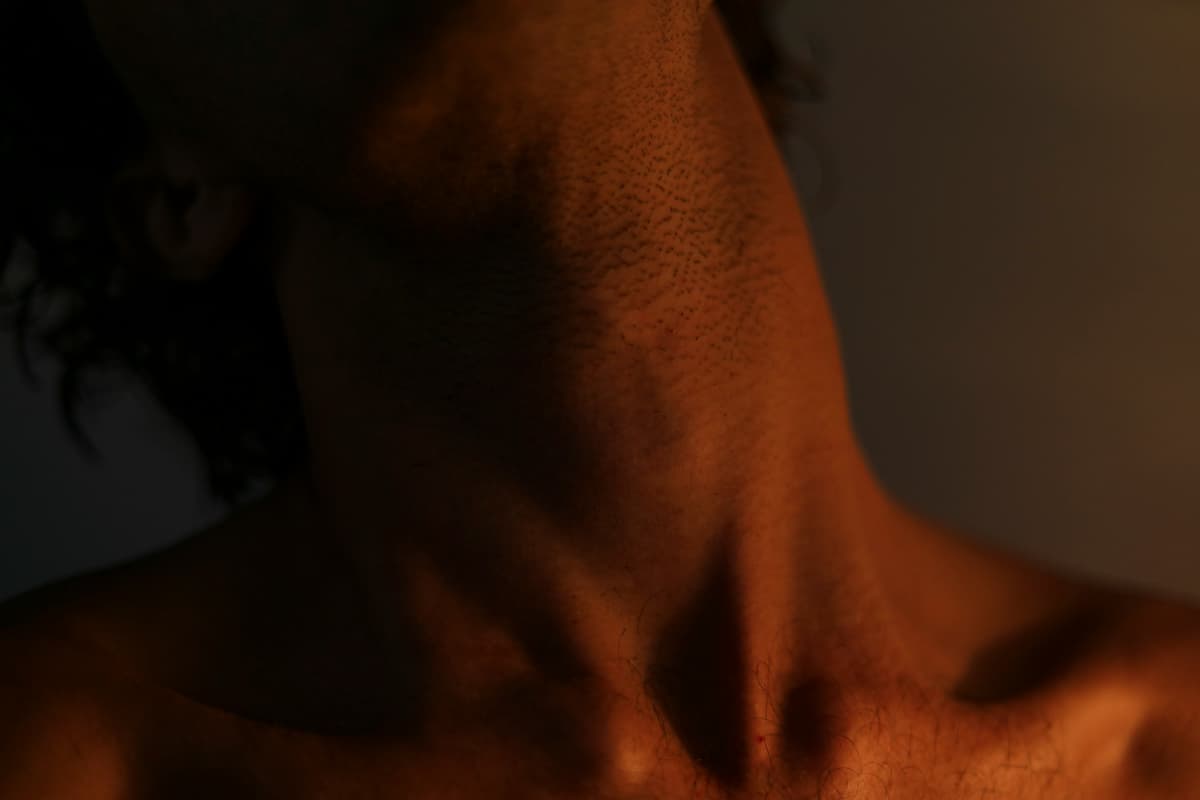
"No reviews yet"
Free
About
Assessing Thyroid Cancer Risk Through Key Health Indicators
Description
Thyroid cancer is a significant health concern globally, and early risk assessment is crucial for effective diagnosis and intervention. This dataset contains 212,691 records detailing demographic information, clinical history, lifestyle factors, and thyroid hormone levels. It is ideal for developing machine-learning models to predict thyroid cancer risk and exploring correlations between health indicators and cancer diagnosis.
Dataset Features
Each record corresponds to a patient and includes the following attributes:
- Patient_ID (int): Unique identifier for each patient.
- Age (int): Patient's age.
- Gender (object): Gender of the patient (Male/Female).
- Country (object): Patient’s country of residence.
- Ethnicity (object): Patient’s ethnic background.
- Family_History (object): Presence of thyroid cancer in the family (Yes/No).
- Radiation_Exposure (object): History of radiation exposure (Yes/No).
- Iodine_Deficiency (object): Presence of iodine deficiency (Yes/No).
- Smoking (object): Whether the patient smokes (Yes/No).
- Obesity (object): Whether the patient is obese (Yes/No).
- Diabetes (object): Whether the patient has diabetes (Yes/No).
- TSH_Level (float): Thyroid-Stimulating Hormone level (µIU/mL).
- T3_Level (float): Triiodothyronine level (ng/dL).
- T4_Level (float): Thyroxine level (µg/dL).
- Nodule_Size (float): Size of thyroid nodules (cm).
- Thyroid_Cancer_Risk (object): Estimated risk level (Low/Medium/High).
- Diagnosis (object): Final diagnosis (Benign/Malignant).
Distribution
- Data Format: CSV (or Excel)
- Data Volume: 212,691 records and 17 columns
- Data Structure: Tabular format with a mix of numerical and categorical features
Usage
The dataset supports a wide range of applications:
- Exploratory Data Analysis (EDA): Identify patterns and visualize relationships between lifestyle factors and thyroid cancer risk.
- Predictive Modeling: Train machine-learning models to classify patients by their thyroid cancer risk.
- Healthcare Analytics: Build dashboards to assist clinicians in thyroid cancer risk assessment.
- Deep Learning: Apply advanced neural networks for complex pattern detection and risk evaluation.
- Statistical Testing: Perform correlation analysis to evaluate relationships between health factors and thyroid cancer occurrence.
Coverage
- Geographic Coverage: Diverse patient population across multiple countries.
- Time Range: Not specified.
- Demographics: Includes age, gender, ethnicity, and clinical history.
License
- License: CC0 (Public Domain)
Who Can Use It
This dataset is beneficial for:
- Data Scientists: For developing predictive models for thyroid cancer detection.
- Healthcare Professionals: To assess patient risk and improve diagnostic accuracy.
- Researchers: For studying the correlation between thyroid-related indicators and cancer outcomes.
- Students: To learn classification techniques and apply statistical analysis on real-world health data.
This dataset provides a robust foundation for advancing thyroid cancer research, developing clinical support tools, and improving early detection methods.