Heart Attack Prediction Dataset
Public Health & Epidemiology
Related Searches
Trusted By
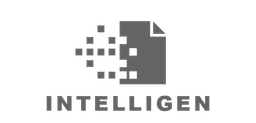
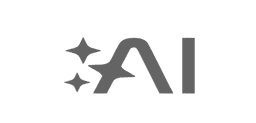
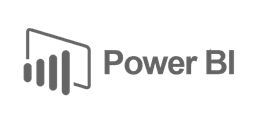
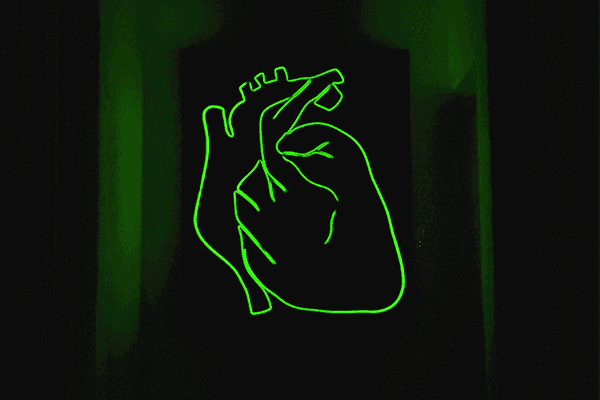
"No reviews yet"
Free
About
Designed to support the prediction of heart disease likelihood in patients, this dataset includes 14 critical health and medical attributes selected from an original set of 76 features. Widely utilized in medical machine learning research, it enables binary classification to determine a patient’s risk of experiencing a heart attack.
Features:
- Age: Age of the patient (years).
- Sex: Gender of the patient (1 = male, 0 = female).
- Chest Pain Type: Type of chest pain experienced (values range from 0 to 3, representing different types of chest pain).
- Resting Blood Pressure: Resting blood pressure (mm Hg on admission to the hospital).
- Cholesterol: Serum cholesterol in mg/dl.
- Fasting Blood Sugar: Fasting blood sugar level > 120 mg/dl (1 = true, 0 = false).
- Resting ECG Results: Resting electrocardiographic results (values 0, 1, 2).
- Max Heart Rate Achieved: Maximum heart rate achieved during exercise.
- Exercise Induced Angina: Exercise-induced chest pain (1 = yes, 0 = no).
- Oldpeak: ST depression induced by exercise relative to rest.
- Slope of Peak Exercise ST Segment: The slope of the peak exercise ST segment (values 0, 1, 2).
- Number of Major Vessels: Number of major vessels colored by fluoroscopy (values 0-3).
- Thalassemia: Thalassemia blood disorder status (values 1 = normal, 2 = fixed defect, 3 = reversible defect).
- Target: Indicates the risk of heart disease (0 = low/no chance, 1 = higher chance).
Usage:
This dataset is an excellent resource for:
- Binary classification tasks in machine learning to predict heart disease.
- Developing models to assist in early detection and intervention for patients at risk.
- Conducting feature analysis to identify key factors contributing to heart disease.
Coverage:
The dataset is derived from multiple clinical studies and includes anonymized patient data. It’s widely used in medical research and machine learning applications.
License:
CC0 (Public Domain)
Who can use it:
Healthcare data scientists, machine learning practitioners, and researchers focused on cardiovascular health can benefit from using this dataset for predictive modeling and feature exploration.
How to use it:
- Build and evaluate classification models, such as logistic regression, decision trees, or neural networks, to predict heart disease risk.
- Use feature importance techniques to identify the most significant factors in heart disease prediction.
- Apply the dataset to healthcare applications that support medical diagnostics and preventive care strategies.