Cancer Prediction Dataset
Patient Health Records & Digital Health
Related Searches
Trusted By
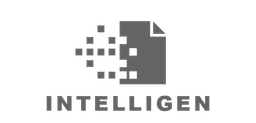
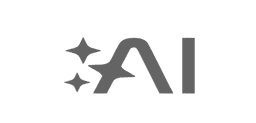
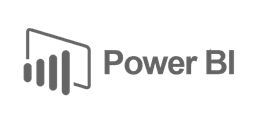
.jpg&w=1200&q=75)
"No reviews yet"
Free
About
This dataset includes medical and lifestyle information for 1,500 patients. It is designed to predict whether someone has cancer-based on various Features. The dataset provides a realistic challenge for creating predictive models in healthcare.
Dataset Features:
- Age: Patient's age in years, ranging from 20 to 80.
- Gender: Patient's gender, classified as male or female.
- Body Mass Index (BMI): A continuous value indicating body fat based on height and weight, ranging from 15 (underweight) to 40 (obese).
- Smoking Status: Indicates if the patient is a smoker (Yes or No).
- Genetic Risk: Evaluates hereditary health influences as Low, Medium, or High risk.
- Physical Activity: Time spent on physical activities per week, measured in hours (0 to 10).
- Alcohol Intake: Weekly alcohol consumption, ranging from 0 to 5 units.
- Cancer History: Indicates personal history of cancer (Yes or No).
- Diagnosis: States whether the patient has been diagnosed with a condition (Yes or No).
Usage:
The dataset is ideal for training and testing machine learning models in cancer prediction. Potential applications include:
- Training and evaluating predictive models.
- Analysing feature importance in cancer prediction.
- Benchmarking machine learning algorithms in a healthcare context.
Coverage:
The dataset covers key demographic, lifestyle, and genetic risk factors associated with cancer risk, supporting a broad range of modelling approaches and feature engineering techniques.
License:
CC0 (Public Domain)
Who can use it:
This dataset is intended for data scientists, machine learning practitioners, researchers, and students interested in exploring healthcare predictive analytics.
How to use it:
- Develop predictive models, conduct feature analysis, or compare different algorithms.
- Investigate the relationships between mental health, study habits, and academic performance through correlation analysis.
- Analyse trends in mental health factors across demographics within the student population through data exploration.