Synthetic Diabetes Prediction Dataset
Patient Health Records & Digital Health
Related Searches
Trusted By
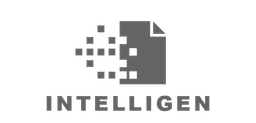
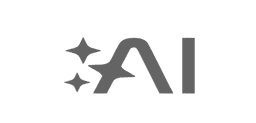
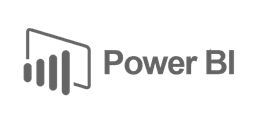
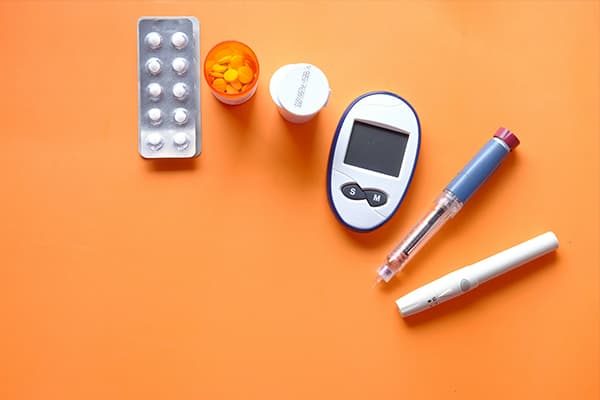
"No reviews yet"
£179.99
About
The Synthetic Diabetes Prediction Dataset is designed for educational and research purposes to analyze health-related factors contributing to diabetes risk. It provides anonymized, synthetic data on individuals’ demographic information, medical history, and lifestyle choices.
Dataset Features
- gender: Gender of the individual (Male/Female).
- age: Age of the individual (in years).
- hypertension: Presence of hypertension (Yes/No).
- heart_disease: Presence of heart disease (Yes/No).
- smoking_history: Smoking history (e.g., never, formerly, current, No Info).
- bmi: Body Mass Index (BMI) of the individual.
- HbA1c_level: Hemoglobin A1c level (a measure of blood sugar over time).
- blood_glucose_level: Random blood glucose level (mg/dL).
- diabetes: Diabetes diagnosis (Yes/No).
Distribution
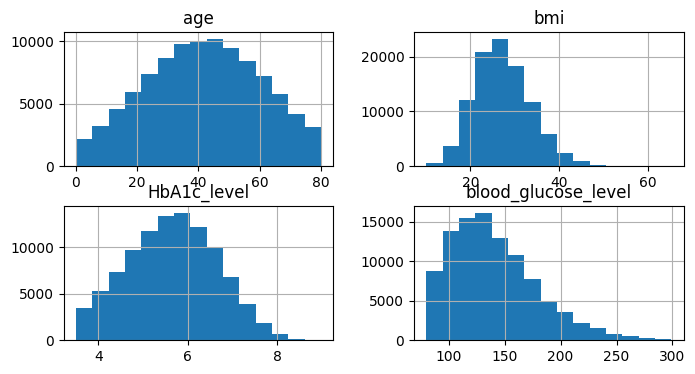
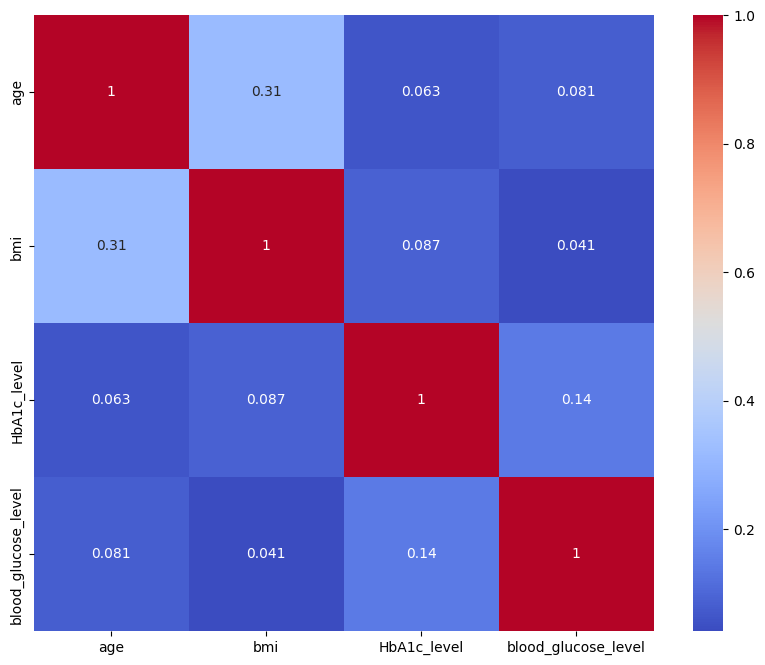
Usage
This dataset can be used for the following applications:
- Diabetes Risk Prediction: Build models to predict diabetes status based on demographic, medical, and lifestyle factors.
- Health Behavior Analysis: Study the impact of BMI, smoking history, and other health indicators on diabetes prevalence.
- Medical Research: Explore correlations between hypertension, heart disease, and diabetes.
- Preventative Healthcare: Identify key predictors to inform early detection and prevention strategies for diabetes.
- Policy and Decision Making: Provide insights into population health to guide public health initiatives.
Coverage
This synthetic dataset is anonymized and adheres to data privacy standards. It represents diverse demographics and health profiles, enabling broad applications in healthcare research and analysis.
License
CC0 (Public Domain)
Who Can Use It
- Data Scientists and Machine Learning Practitioners: For classification tasks related to diabetes prediction and risk analysis.
- Healthcare Researchers: To study risk factors and prevalence of diabetes in a synthetic population.
- Public Health Professionals: For insights into population health trends and preventative care.
- Educators and Students: As a teaching resource for analyzing health-related datasets and building predictive models.