Synthetic Heart Disease Dataset
Clinical Trials & Research
Related Searches
Trusted By
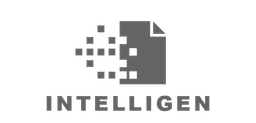
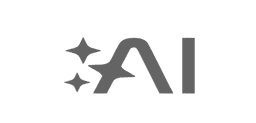
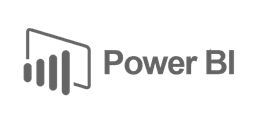
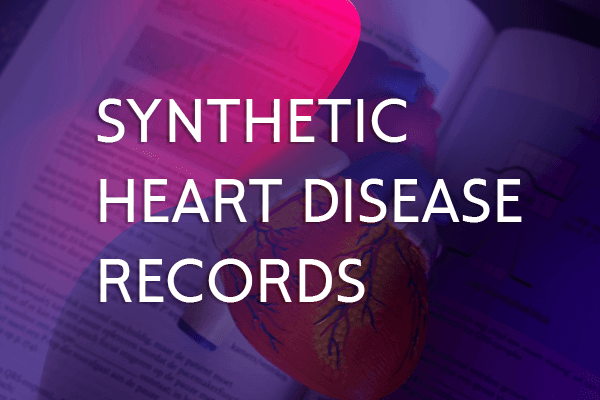
"No reviews yet"
£45.99
About
A synthetic heart disease dataset has been generated to serve as an educational resource for data science, machine learning, and data analysis applications in the healthcare industry. It simulates patient records related to heart disease, allowing users to practice data manipulation and develop analytical skills in a healthcare context.
Dataset Features:
- Age: Age of the patient at admission (in years).
- Country: Country of residence, specified as the USA.
- State: Random assignments of U.S. states for geographic analysis.
- Blood Pressure: Simulated values reflecting typical hypertension ranges (in mmHg).
- Cholesterol: Values adjusted to fall within common cholesterol levels (in mg/dL).
- BMI: Calculated to represent healthy to overweight classifications.
- Glucose Level: Simulated to represent fasting glucose levels (in mg/dL).
- Gender: Randomly assigned to simulate demographic diversity.
- Hospital: Randomly assigned hospitals to represent different healthcare facilities.
- Treatment Options: Various treatment methods including Physiotherapy, Medication, Surgery, Rehabilitation, and Counseling.
- Treatment Date: Randomly generated dates for when treatments were administered.
- Heart Disease: A binary indicator (0 = No, 1 = Yes) representing the presence of heart disease.
Data Distribution and Outliers:
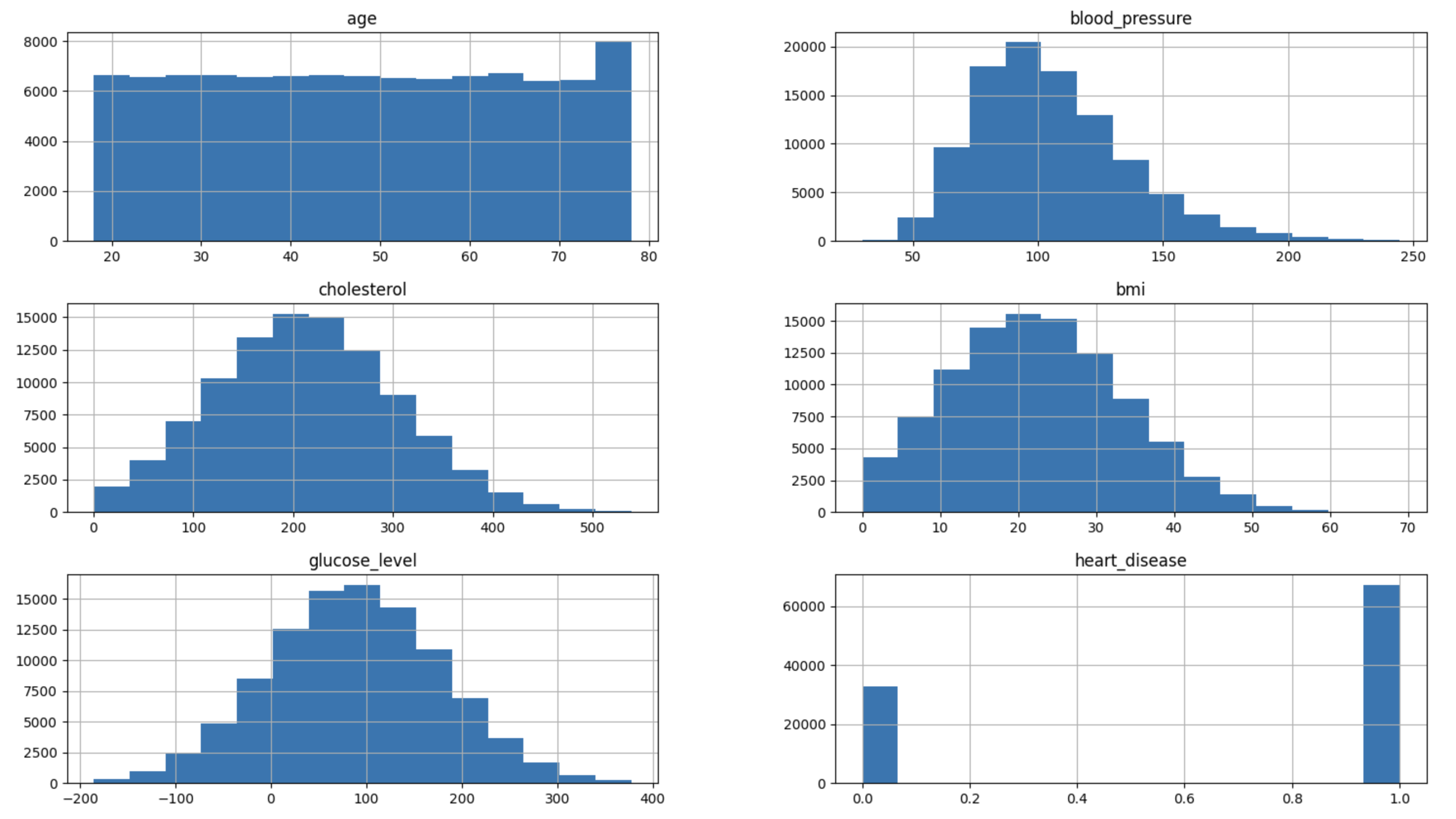
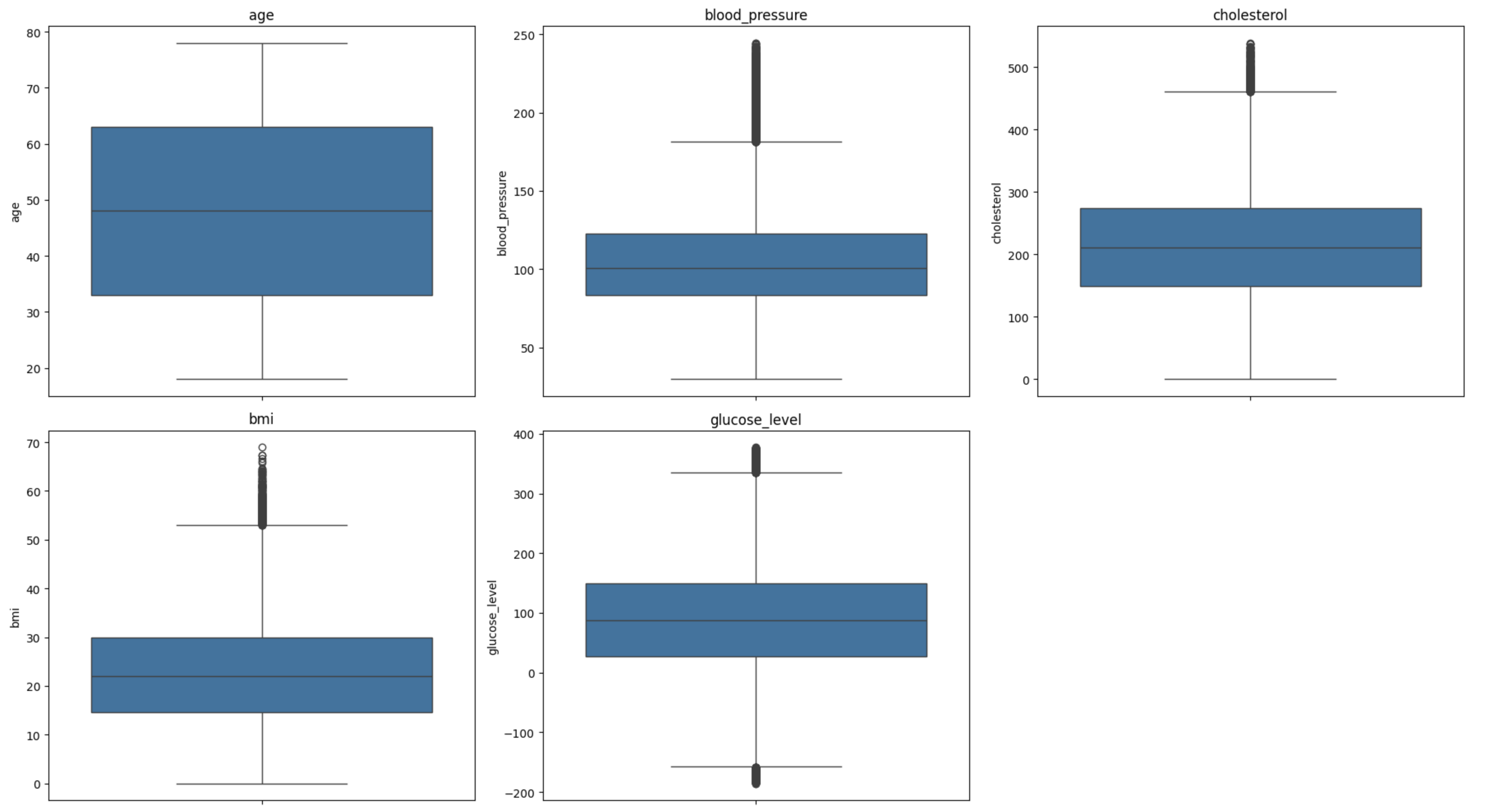
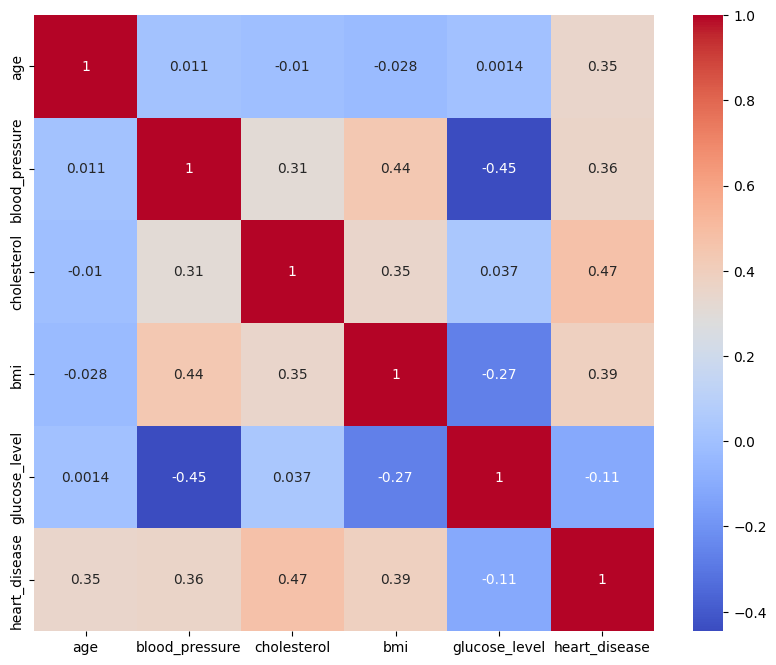
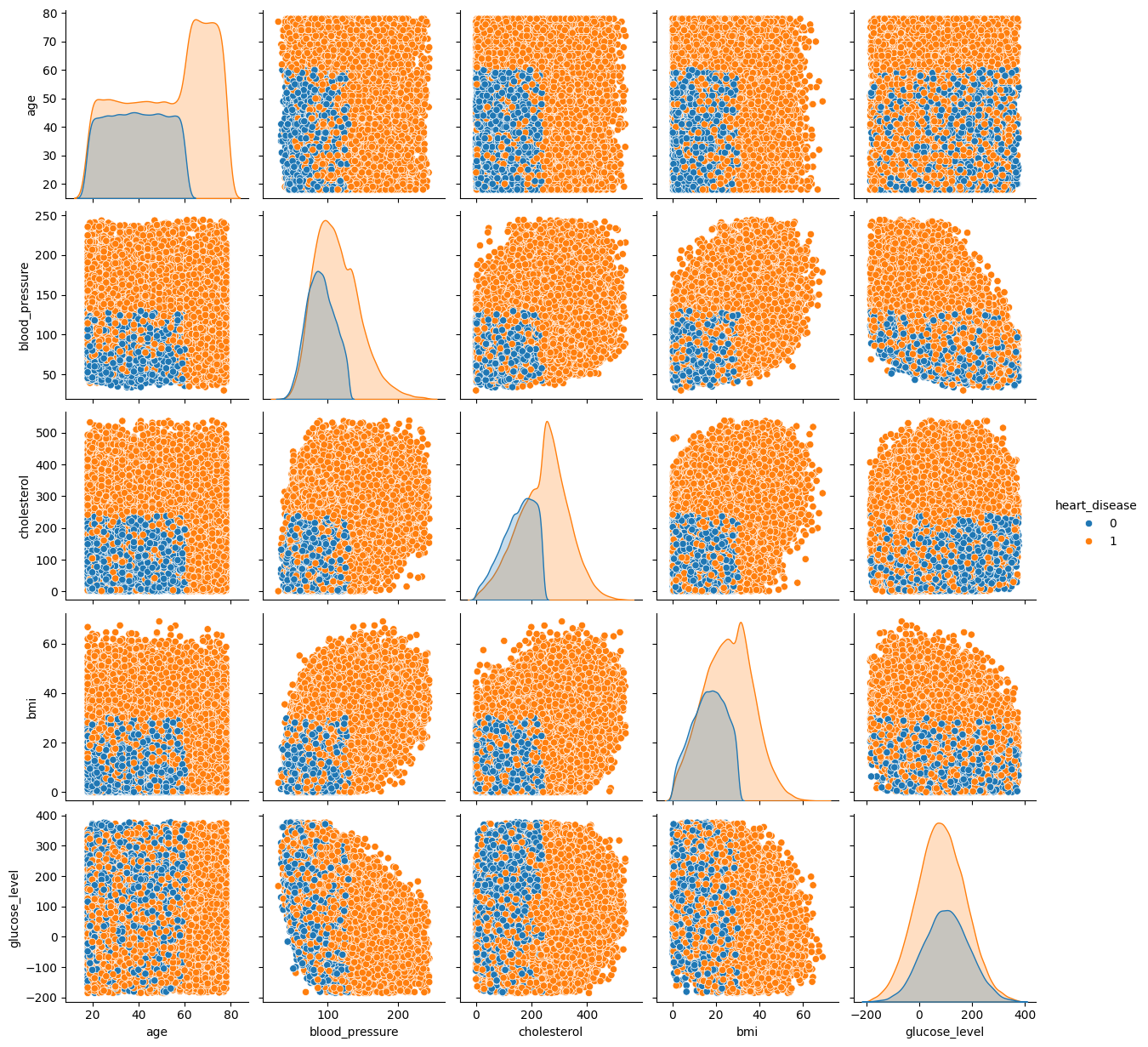
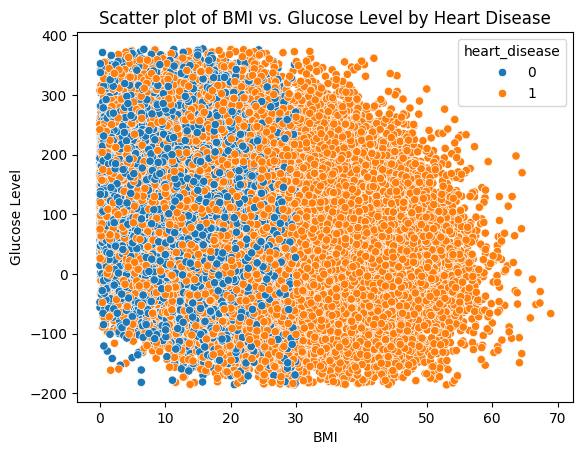
Usage:
This dataset can be used for:
- Healthcare research: To explore trends and patterns in cardiovascular health, treatment efficacy, and patient demographics.
- Educational training: To teach data cleaning, transformation, and visualisation techniques specific to healthcare data.
- Predictive modelling: To develop models that predict heart disease risk based on various patient and demographic factors.
Coverage:
This dataset is synthetic and anonymized, making it a safe tool for experimentation and learning without compromising real patient privacy.
License:
CCO (Public Domain)
Who can use it:
- Researchers and educators: For studies or teaching purposes in healthcare analytics and data science.
- Data science enthusiasts: For learning, practising, and applying healthcare data manipulation and analysis techniques.